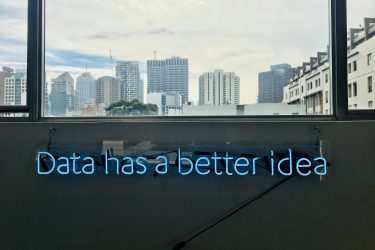
How can EMNLP 2021 help to shape reality? On language models, stereotypes and biases mitigation at 360°
Why is Natural Language Processing (aka NLP) – and conferences like EMNLP 2021 – important for our society? As a journalist, I often find myself struggling while tying to explain to my colleagues how Distributional Semantics (DS) Language Models work. In my view it’s self explanatory that communication experts – as they claim to be – should best know which shape language takes after having been computationally formalized, e.g. which biases entails the process of words (or sentence or text) vectorization and why, or how to provide for better language representations etc. By the way, this is the reason why I am now studying ML&NLP at Cimec in Rovereto (Italy). At the latest with the famous paper on “male brilliance” by (Leslie et al. 2015) should have become clear to everyone that we, as human beings, are biased – evolution provided us with those mostly useful mental shortcuts – and, as a consequence, also language contains biases. Journalists (at least those who care about it) want to shape a better world through more equal, non categorical representations. But in everyday life, then, they use biased search engines to browse the web or look for pictures for their articles, or they are targeted by biased suggestions popping up in the ads, etc. Though they mostly don’t question their instruments and methods at all.
Photo by AbsolutVision on Unsplash
EMNLP 2021 gives a great opportunity to an insight on this issue. In the first day of the conference a whole panel was devoted to Ethics and NLP. Thanks to the Semantic Paths by University La Sapienza NLP, the multilingual semantic search engine for EMNLP 2021, it isn’t even necessary to master Topic Modeling to find out how many papers and authors at this EMNLP edition have dealt with this issue. And from the most different perspectives: look for example for papers related to “sexism”, “algorithmic bias”, “decision making”, “confirmation bias”, only to mention a few. Image search is maybe the field where biases emerge in the most striking way. In their paper “Are Gender-Neutral Queries Really Gender-Neutral? Mitigating Gender Bias in Image Search“, Jialu Wang, Yang Liu and Xin Wang study a unique gender bias in image search, i.e. the search images are often gender-imbalanced for gender-neutral natural language queries. The proposed two novel debiasing approaches show a reduction of the gender bias on MS-COCO and Flickr30K benchmarks. Also some more methodological approaches are to find, like the one in the paper “Modeling Disclosive Transparency in NLP Application Descriptions“, where Michael Saxon, Alon Albalak, Sharon Levy, William Yang Wang, Xinyi Wang: a claim for more transparency in describing NLP applications.
Slide from the paper: “Are Gender-Neutral Queries Really Gender-Neutral? Mitigating Gender Bias in Image Search”
Also Q&A sessions face the issue. The paper “WIKIBIAS: Detecting Multi-Span Subjective Biases in Language” by Yang Zhong, Wei Xu, Diyi Yang, Jingfeng Yang investigate subjective bias – a special type of bias that introduces improper attitudes or presents a statement with the presupposition of truth – by means of a manually annotated parallel corpus WIKIBIAS with more than 4,000 sentence pairs from Wikipedia edits. The issue has been tackeld also from the perspective of semantics during the Sunday November 7 session on Semantics by the paper of by Rochelle Choenni, Ekaterina Shutova and Robert van Rooij “Stepmothers are mean and academics are pretentious: What do pretrained language models learn about you?“. The study investigate stereotypical information captured by pre-trained language models. by means of a dataset comprising stereotypical attributes of a range of social groups. This allows to study how stereoptypes are encoded in language models and how quickly emotions – as manifestations of those emergent stereotypes – can shift at the fine-tuning stage.
Slide from the paper: Stepmothers are mean and academics are pretentious: What do pretrained language models learn about you?